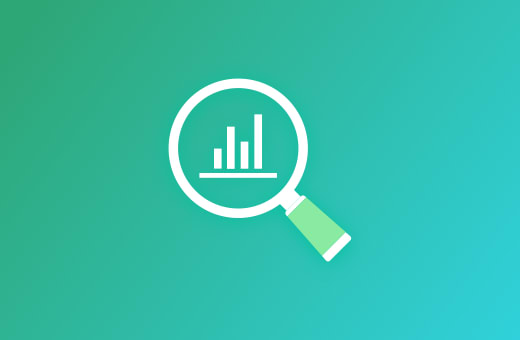
Data And Data Analysis
Data And Data Analysis
Psychological research often requires a numerical and quantitative organization of results that they get from their findings – the results in question is called ‘raw data’. To categorize large findings in these scenarios, it is often mathematically simplified and visually represented via graphs.
Quantitative Data And Qualitative Data:
The numerical results collected by psychologists is known as ‘quantitative data’, the data which is detailed and descriptive is called ‘qualitative data’. Quantitative data indicates the quantity of the psychological measure I.e. the strength or amount of a response and tends to be measured on scales, such as time, or as numeric score on tests such as Personality, IQ and T-maze tests.
Quantitative data is associated with experiments and correlations which use numeric scales but it is also possible to collect quantitative data from observations and interviews.
Quantitative Data | Qualitative Data |
Strengths:
Weaknesses:
| Strengths:
Weaknesses:
|
Example:
An example of quantitative data would be a total number of responses recorded of a certain behaviour (such as a variation of facial expressions on a scale) during an interview.
The sources of quantitative data are usually stictly objective as the measure used (closed questionnaires, scales, etc) don’t really need much interpretation. These measures are thus reliable.
An example of qualitative data would be a verbal account of an incident that the participant gives in an interview. The data is more in-depth. This is gathered via open-question questionnaires, interviews and case studies. This while does make the data subjective and difficult to interpret, it is still valid in terms of being accurate in the aim.
Data Analysis:
Measures of central tendency:
A set of quantitative results can be boiled down to represent the middle score and an aggregate – this is known as measures of central tendency.
The Mode:
The mode is most the frequently repeated score, number in a data set. There can be more than 2 modes. It is unaffected by extreme scores and it is useful to observe repetitive behavioural patterns. An example of this would be Milgram’s study where there was a modal value of the voltage scale that was frequented on participants.
Limitations of using this measure is that it offers no insight about the other scores, it isn’t very ‘central’, it is also very fluctuating from one sample to another.
Example:
545350,8,7,7,7,7,0. - the mode is 7.
The Median:
Unlike the mode, the median cannot be used with data indiscrete categories as it is only used with numerical data on a linear scale. To find the median, all the scores in the data set are put in a list from smallest to the largest. The middle one in the list is called the ‘median’. To configure this, all scores are arranged from ascending order – the middle number in this is the median value. If there are an even number of participants, in which case there are two numbers in the middle, these are added together and then divide by 2.
In essence, the median value is the halfway point that separates the lower quartile from the upper quartile. It is unaffected by extremes, in the sense that there is no distortion of results. It however can be misleading when there are only a few scores and doesn’t take into account most of them.
Example:
Score set: 3,4,7,8,9,0,2,2,6 – median being 9.
Score set: 2,6,8,10,13,16 – median being = 8+10/2 = 9.
Mean:
The mean is the measure of central tendency that we usually call the ‘average’. It can only be used with numerical data from linear scales. The mean is worked out by adding up all the scores in the data set and dividing them by the total number of scores. It is the most thorough and informative measure of central tendency as it takes into account all scores. There is however the probability of it giving a distorted result if there are any anomalous scores.
It is done by adding up all the values to find a total, dividing the value by the number of values added together that were present.
Example:
2+4+6+8+10+12/6= 7 – the mean value.
Measures of Spread
This indicates how far spread, dispersed and varied data is within a set. If two data sets are the same size, with the same mean, they could still avry in terms of how close the majority of data points were to that average. Differences such as this are described by measures of spread: the range and the standard deviation.
The range:
It is the simplest measure of spread.
To calculate:
1. Find the largest and smallest value in the set of data.
2. Subtract the smallest value from the largest value and add 1.
Conventionally, the addition of 1 is not done. In psychological research this is done so that we measure the gaps between points, not the points themselves.
The Standard Deviation:
In the same way that the mean can tell us more than the mode, a measure of spread called the standard deviation can tell us more than the range. Range than looking only at the extremes of the data set, the standard deviation takes into account the difference between each data point and the mean – this is known as deviation from the standard.
As the standard deviation tells us the spread of a group, groups with scores that are more widely dispersed have a larger standard deviation. When the standard deviations of two groups are similar, this indicates they have a similar variation around the mean/average.
Graphs:
This is used to visually illustrate data, with a variety of them for different purposes. The ones being included in our syllabus being Bar charts, Histograms and scatter graphs.
Bar Charts:
A bar chart is used when data is in separate categories rather than a continuous scale. Bar charts are therefore used for the totals of data collected in named categories and for all measures of central tendency.
The bars on a bar chart must be separate. The x-axis represents distinct groups of values (such as the DV) and not a linear scale. For an experiment – the IV levels are put on the Y-axis while the DV levels are put on the x-axis.
Histograms:
Histograms are useful to show the pattern in whole data set, where the data is continuous in which case the data is being measured on a scale rather than distinct categories. A histogram may be used to illustrate the distribution of a set of scores. In this case, the DV is plotted upon the x-axis (these may be grouped categorically) while the frequency of each score is plotted along the y-axis. As a scale represented on the x-axis is continuous the bars are drawn next to each other, with no gap. Thus, if a category has no score, it must be left to shown as empty.
Scatter Graphs:
The results which are collected from a correlational study are presented on a scatter graph. To construct a scatter graph, a dot is marked at a point where the participant’s score on each variable cross, there is also the ‘line of best fit’ reoccurring on a scatter graph. The position of this line is calculated and its line is drawn so that it comes close to as many ‘points’ as possible. In the case of a strong correlation, all the data points lie near/close to the line whereas in a weak correlation’ its vice versa – they are more spread out. When there is no correlation, a concrete line is not formed.
It is also a significant aspect to keep in mind that correlation does mean causation; we cannot determine causality from the findings. Scatter graphs thus, only do so much as to explore and tell us about the relationship between variables. An experiment could help us find cause however.